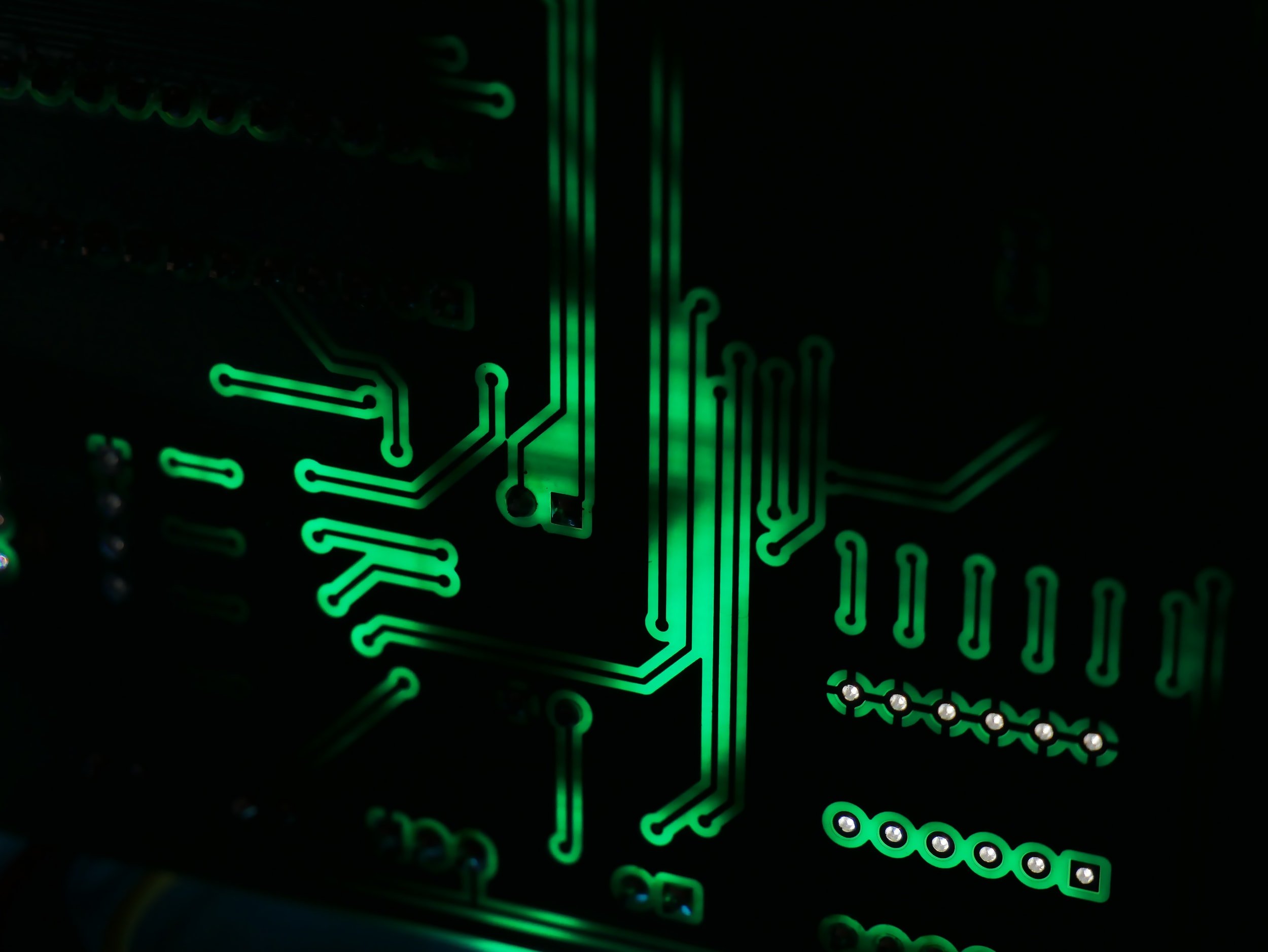
Carbon trading
PRICE FORECASTING
Over the last 4 years we have developed a suite of proprietary models for the accurate forecasting of energy and environmental asset prices over various timeframes.
For example, we have both multi-day (‘Micro’) and multi-month (‘Macro’) price forecasts for “EU EUA” allowances (which represent the right for a Covered Entity to emit 1 ton of CO2-equivalent GHGs within the European Union’s Emissions Trading Scheme (ETS)).
Forecasts using local data sets combined with our macro data sets can be provided for any national ETS.
EU ETS EUAs are actively traded on both spot and futures markets. EU ETS “Covered Entities” need to ensure they purchase the right quantity to match their year’s carbon emissions. The market price varies over time, so there is scope to save money by optimising one’s timing in the market.
Financial traders such as commodity trading funds, hedge funds, investment banks and brokers and similar can also utilise price forecasting to achieve higher portfolio returns.
Additionally, the EUA price will determine the EU Carbon Border Adjustment Mechanism charge (to buy certificates) which will be payable to the EU when importing 1 ton of in-scope product (e.g. steel, ammonia etc) into the EU.
PROCESS
• The model provides a general innovative method with base learners to predict carbon and oil time series monthly data in high dimensions. In particular, we innovate on real time to improve predictive accuracy.
• We utilise an ensemble learning technique that combines multiple predictive learner models and allows for the additions of other learners.
• different algorithms generate varying predictions on the same data because characteristics (and covariates) are allocated different levels of importance by each algorithm. The evolution over time of the data and the economic and market environments are thus captured in the event that some fundamental structure changes occur.
• by dynamically and optimally combining multiple algorithms for each observation, we are able to adapt to new data and improve the predictive accuracy in a walk-forward manner.
• the decomposition method uses pattern detection techniques to decomposes the original series into components. It can purge non-stationary trends from the prices, leaving the most volatile stationary part for the learners to handle.
ACTUAL PERFORMANCE
Short Term (daily) carbon price (EUA) prediction accuracy: 83.1% directional accuracy with 2.5% error (RMSE)
Long Term (3 months to 18 months) carbon price (EUA) prediction accuracy: 81.5% directional accuracy with 8.1% error (RMSE)
actual achieved trading results average return 61.8% per quarter (CAGR 585.4%)
Note of course that past results are not necessarily a good guide to future results.
The algorithm can also be used for predicting oil (Brent and WTI) prices and other commodity prices.
This sort of forecast, and the ‘cost of carbon’ it represents, can also be integrated into more complex financial analyses, for example the payback period for a proposed investment project.
CONTACT FOR MORE INFORMATION
Contact connect@climatecap.net for more information and/or bespoke analysis.